
Zo helpt JUVO jou
Jou helpen om méér te doen met jouw data. Zodat jij, gedreven door data, stappen vooruit zet. Dat is de opzet. En dat doen we op drie onafhankelijke niveaus.
Enable
Wat wil je bereiken? Welke data heb je? Hoe zet je die in?
JUVO zorgt ervoor dat je jouw data slim inzet. Zodat je jouw doelen behaalt en nog beter wordt in wat je doet.
Optimize
Door out-of-the-box te denken en je IT-architectuur anders te bekijken, krijg je nieuwe inzichten. Optimize koppelt je data aan je business. Zo haal je nog meer uit jouw data en til je je bedrijf naar een hoger niveau.
60+ tevreden klanten
4,6/5
Inventief gebruik van data geeft je een competitief voordeel. Klanten waarderen JUVO omdat we hen helpen om hun data anders te benaderen. En op een andere manier in te zetten. We zien data als een krachtig middel om je doelen te halen.
Onze basispricipes
- Sharing is caring
- Open en direct
- Loyaal en betrouwbaar
- We love challenges
Dexis Belgium
Dexis Belgium verhoogt zijn klantenservice, én de ondersteuning van zijn medewerkers. Door meer inzicht in het businessproces en modernisering van zijn business intelligent tool.

Port of Antwerp
Port of Antwerp maakt slim gebruik van data om de werking van de haven in goede banen te leiden.
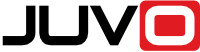
Over Juvo
Meer inzicht in jouw data, een beter begrip en een hoger rendement. JUVO koppelt jouw data aan je business zodat jij (sneller) kunt groeien.
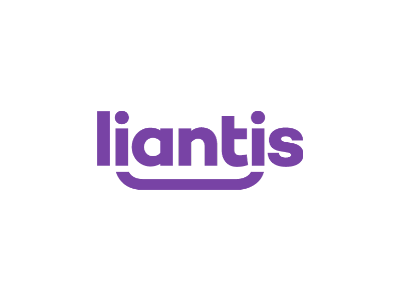
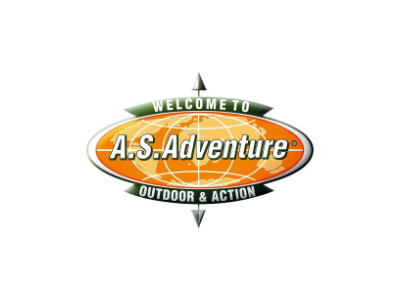

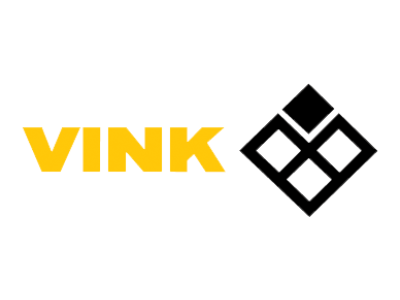
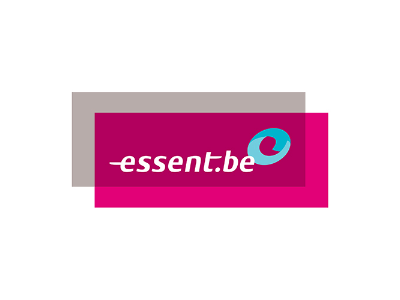
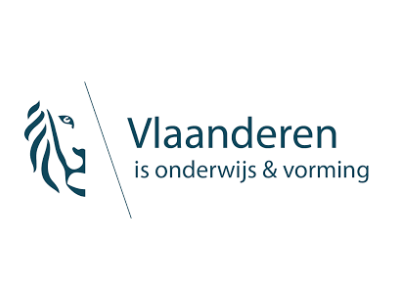
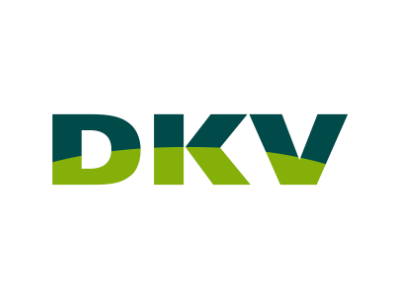
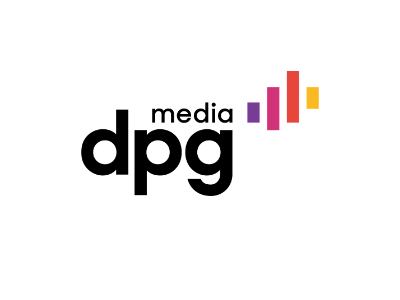
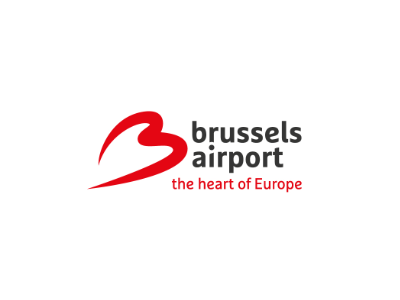
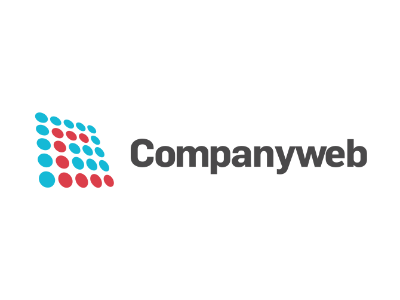

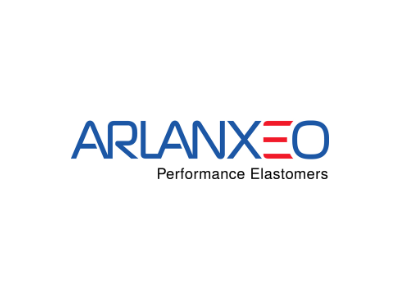
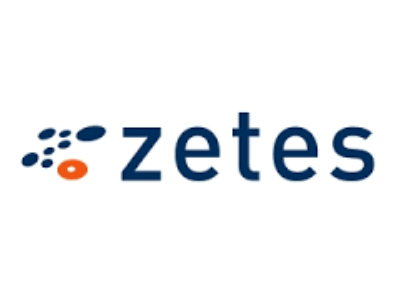
Hecht team experts
Data-experts die kennis delen met elkaar en inzetten om klanten vooruit te helpen. Gebeten door data en altijd geïnteresseerd in nieuwe technologieën. Dat zijn de JUVO-consultants.
Werken bij Juvo?
Wil jij erbij horen? Bekijk onze vacatures. En staat de job van je dromen er niet tussen? Ook jouw spontane sollicitatie ontvangen we met open armen.
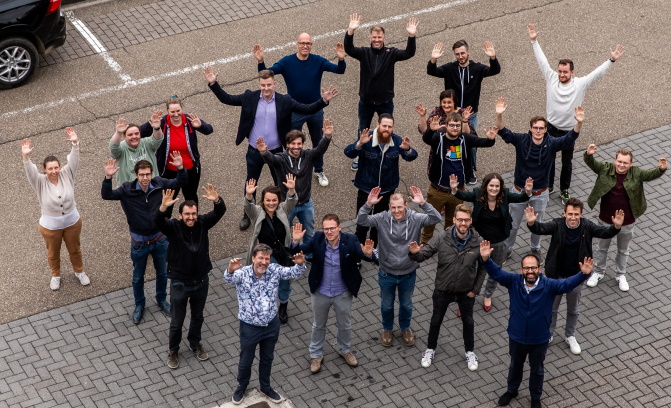
Haal meer uit je data met gratis webinars
Zelf Efficiënt aan de slag met jouw data
Samenwerken met Juvo
Met welke oplossingen start je?
Dat ligt eraan wat je nodig hebt. Afhankelijk van jouw behoefte ga je voor enable, build of optimize. De drie oplossingen zijn onafhankelijk van elkaar inzetbaar.
Hoe werken we samen?
Dat kies je zelf. Heb je extra expertise nodig op een project? Een applicatieontwikkelaar om je processen te automatiseren? Een data engineer om meer uit je data te halen? Een klankbord om je ideeën af te toetsen? Of een projectmanager die jouw project in goede banen leidt? Alles is mogelijk. We bespreken het graag met je.
Hoe helpen we jou?
Door naar je te luisteren, onze expertise voor je in te zetten en ervoor te zorgen dat jij uit je data alles haalt wat erin zit. We maken analyses en ontwikkelen de applicaties die je daarvoor nodig hebt.
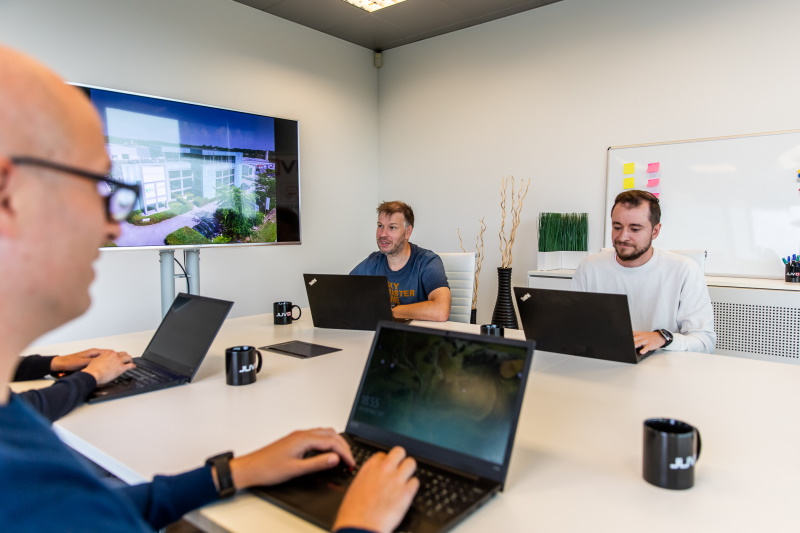